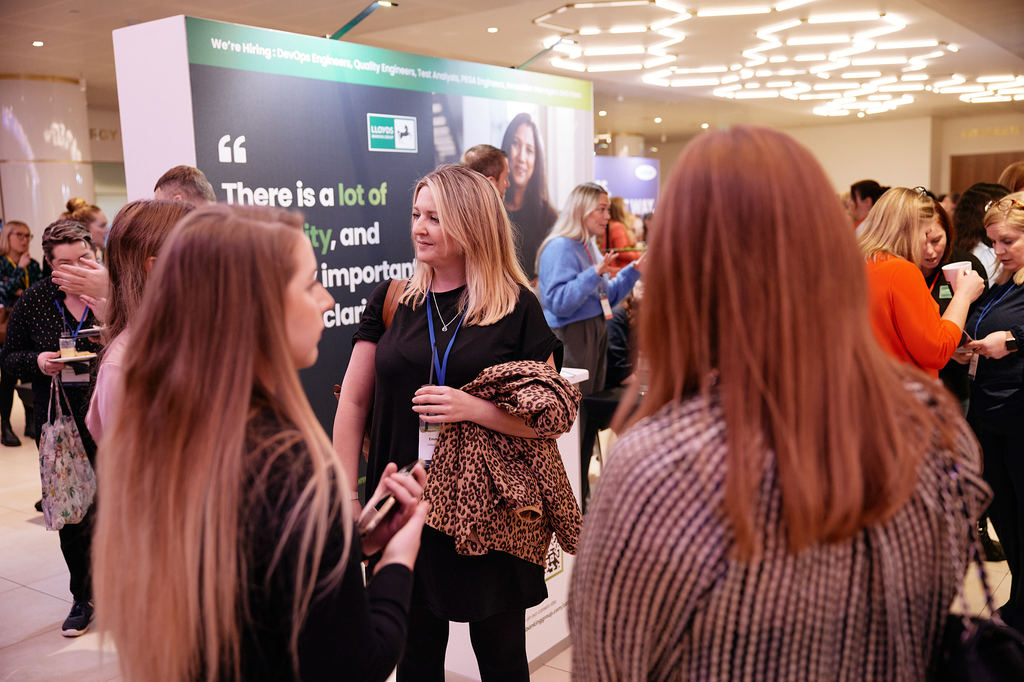
Back Diversity data: a problem, and a solution
Diversity data: a problem, and a solution
Amid the attention on exciting developments in AI and its potential for sophisticated data analysis, a common theme in commentary is that no matter how clever this technology is, your outputs are only as good as your inputs. Get these wrong, and you risk embedding existing assumptions and biases. Get them right, and you enhance your outputs – such as insights that guide impactful action – across the board.
This is particularly true with data, and its power to drive diversity in business. Whether in a company’s own operations or their supply chain, the most sophisticated analysis tools are of little use without good, relevant, raw data.
To drive diversity, this means gathering and storing business data in a way that captures distinct attributes of people, places, roles, and activities, so datasets can be analysed by these attributes both in isolation and together. Yet even with the largest underrepresented, or “minority” group in business – women – data-gathering exercises don’t always record or save gender-disaggregated information (data separated into male and female sets).
This leaves us with a gender data gap, and limits any analysis on trends or differences between genders and their experiences at work. As we at Sedex know, this lack of gender-separated data is a particular challenge in supply chain assessment, where women (along with other minority groups such as migrant workers) are often at a higher risk of exploitation.
From power imbalances and harassment to wage gaps and lower union representation, differences between men and women’s experiences at work are well-known . In data on global supply chains from Sedex’s platform, we see that:
- In agriculture, women make up 44% of the workforce but hold just 21% of management positions. This data shows us that women are more likely than men to be in lower-paid roles with less decision-making power, affecting both their current experiences and their ability to drive change for the future.
- In apparel manufacturing, top-producing countries like Bangladesh and India are high-risk for gender inequality. This tells us that female workers in this industry are at high risk of discrimination and other forms of exploitation at work.
The International Labour Organization’s most recent analysis also shows that women are still more likely to occupy “traditionally female roles” at work, such as those related to caring, cleaning or cooking. To address these types of issues, we must first identify them – through gender-segmented data.
If you don’t embed this consideration at the point of data capture and systems input, by the time you realise what you’re missing, it’s often too late. But with this data, businesses unlock focused insights to inform initiatives and actions that can drive real change.
Where in your organisation, or supply chain, are women least represented in management roles? This guides prioritisation on where to focus helping women access leadership positions. Combine contract types with an analysis of diversity characteristics – do women, or a minority group, form a high proportion of temporary or zero-hours contracts? If so, there is an opportunity to help these people have more secure employment.
Without the data to provide a gender, ethnicity or other demographic lens, the smartest AI algorithm can’t tell you if one group is more affected by (or vulnerable to) a particular issue. But with this data – and gathering it consistently – we have both actionable insights and clear evidence of positive impact.
As investors, corporate sustainability laws and consumers begin to look beyond a business’s ESG commitments to scrutinise their performance on sustainability issues, this data enables businesses to demonstrate real progress.
Metrics such as more women in leadership roles, increased participation in worker committees and reduced wage gaps demonstrate tangible improvements – more so than an “output” metric such as the number of people who received some training. This doesn’t show whether anything changed as a result.
A lack of diversity data is a vicious cycle that can perpetuate inequalities, but the great news is, it’s easy to break! Begin by embedding just a few additional diversity fields into data capture processes. As every tech professional knows, once that raw data is there, AI-powered tools make short work of it.
Source: Sedex, Progressing Gender Equality in Agriculture, (2022)
Source: Sedex, Apparel Manufacturing Insights Report (2021) and Sedex’s Radar risk tool (2023)
Source: International Labour Organization, Where Women Work, 2023